How Student-Led Initiatives Are Expanding Access and Equity in AI
From working groups and peer learning to programming a first-of-its-kind ACM conference, students are taking the lead in reshaping the fairness debate
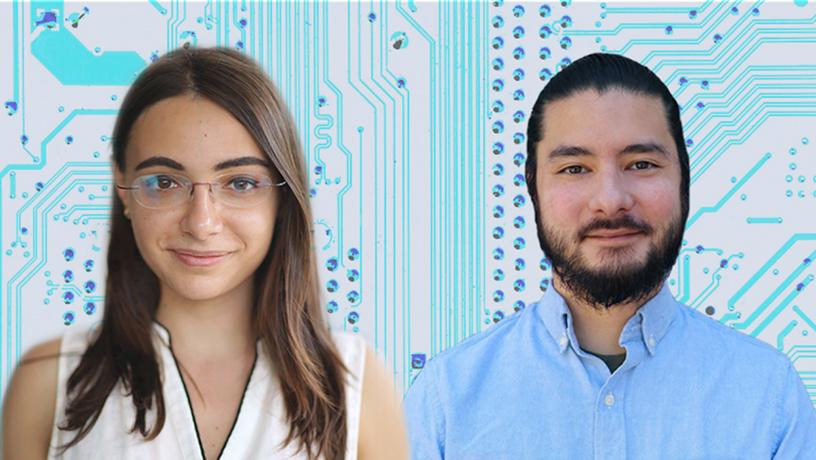
Ana-Andreea Stoica (right) and Roland Maio (left)
By now, many of us have some inkling of the outsize role algorithms have come to play in our daily lives. From recommending which movie to watch to determining whether you can get a home loan, artificial intelligence is ubiquitous, shaping our experiences not only in the digital realm but also in the physical world.
As awareness has grown, so have concerns, about not just about whether we can trust machines to make decisions fairly on our behalf but also how to ensure that computer science—long one of the least diverse STEM fields—proactively leverages its power to create good for all segments of society rather than a select few.
Columbia Engineering magazine caught up with two of the School’s doctoral candidates who are leading significant efforts on this front. Ana-Andreea Stoica, who researches social networks and algorithmic fairness, is a co-organizer of the multinational, interdisciplinary Mechanism Design for Social Good (MD4SG) initiative and a lead on the upcoming inaugural Association for Computing Machinery (ACM) Conference on Equity and Access in Algorithms, Mechanisms, and Optimization. Roland Maio, whose work focuses on fairness and incentives in machine learning and mechanism design, is a co-coordinator of the School’s peer learning Emerging Scholars Program (ESP), a for-credit enrichment program that aims to increase inclusivity and diversity across the undergraduate computer science major by introducing students in lower-level computing courses to advanced topics in the field.
We spoke with Stoica and Maio about how MD4SG and ESP are helping shape broader debates about fairness in computer science and AI. This is an edited excerpt of that conversation.
Q: How do questions of algorithmic fairness connect with your larger research goals?
Ana-Andreea Stoica: I transitioned to computer science after studying math as an undergraduate. I was really fascinated by the different models computer scientists use to try to reproduce dynamics between people. What I found was that in computer science, I was able to both use the technical aspects of those models as well as work on applications that rely on real cases and real data to learn about how we actually connect to each other. In my work, I seek to understand how best to design algorithms with equity and fairness in mind. I’m also continuously learning from other fields to challenge traditional models of computation.
Roland Maio: My research focuses on the interaction between incentives and fairness trade-offs. I wanted to pursue a PhD in computer science because I could see the impact that it was having on society, specifically when it comes to fairness in machine learning. In seeing very clearly that we don’t have all of the answers, at least scientifically, to these problems, I became motivated to become a part of the effort to lay out the scientific contours of the debates that are raging right now and to help inform those debates personally.
One big shift I see is that a lot more junior people are really interested in this field. They’re taking leadership positions, creating these research spaces, and engaging with different communities. And that’s a shift we both saw and created through MD4SG as well.
Q: Ana, you’ve been one of the key organizers of the overall MD4SG initiative. How did you both become involved in MD4SG? What are the group’s larger goals and impact?
AS: At MD4SG, which started as a reading group and has since grown into an organization that spans numerous institutions with over 1,000 members, we organize different working groups with people from different fields, different institutions, and different countries to work on issues related to applications that advance social good. The main mission is to think about using tools to improve opportunity and access to opportunity, and also to identify the right questions that we need to ask. How can we make the computational tools we use more helpful? How can we better understand their limitations? What else do we need to question and change? How can we engage with practitioners in a meaningful way?
For example, we have groups that work on understanding the role of data sharing and data usage in Africa for different computational tools. The development working group is focused on a project to understand problems around vaccine distribution in the Global South and tools for equalizing access to vaccines. The data economies group has been working on a browser for collecting different open datasets from around the world, creating a platform in which data is easily and transparently accessible. We also have a group that works on using computational tools to understand and prevent problems related to the environment and climate change. We’re also trying to do partnerships with different nonprofits that identify issues on which they need research help. Through all of these initiatives, we seek to foster a sense of community between research and practitioners and to engage people from different fields thinking about problems related to social good.
RM: I participated in one of the MD4SG working groups starting last year —actually with Ana— and we worked on a project about trying to bridge machine learning and mechanism design. That resulted in a paper that we submitted to and was accepted by ACM’s Conference on Fairness, Accountability, and Transparency (ACM FAccT). One of the best things about MD4SG is its diversity—for instance, our industry experts bring really interesting experiences and perspectives. And I’m on the program committee and will be reviewing papers for the new ACM conference that Ana is co-organizing, Equity and Access in Algorithms, Mechanisms, and Optimization.
Q: When you look at the growing movement for fairness in computer science, what strikes you about who you see leading that charge?
AS: While more and more there’s an increased awareness that we have to ask the right questions, engage with practitioners and impacted communities, and challenge the way we traditionally think about the tools we use, one big shift I see is that a lot more junior people are really interested in this field. They’re taking leadership positions, creating these research spaces, and engaging with different communities. And that’s a shift we both saw and created through MD4SG as well. The organization has been junior-led, and the different working groups are generally led by graduate students. It’s been amazing to see how these people have really taken ownership of these projects and built a community around them to tackle the problems that they’re interested in.
RM: I’ve always had a sense that computer scientists are idealists who really want the work that they do to have some sort of positive impact on society. And I think there’s been an interesting historical arc whereby the technologies have progressed from being very far removed from political and social issues to being inextricably intertwined nowadays with political and social issues. And for this to be a viable career path, you need to demonstrate the scientific aspect: What are the problems that you’re tackling that actually contribute computational knowledge to society? That is the really incredible milestone and achievement that this conference represents, not just that we have a strong case about the scientific issues to be dealt with here but that it’s being recognized by the entire computer science community.
Q: Roland, you’ve been helping to coordinate the Emerging Scholars Program in Columbia’s Computer Science Department. This program takes the form of a weekly workshop for first- and second-year undergraduates that’s led by juniors and seniors. Can you talk a bit about the pedagogical foundations of peer learning, and why it has enjoyed so much success here?
RM: So, the program can trace its historical pedagogical foundations to two separate peer-learning-based programs: the Emerging Scholars Program that Uri Treisman, now a mathematician at UT Austin, created in the 1970s, and the Peer-Led Team Learning program created in the 1990s at the City College of New York. The Columbia version combines elements of these two programs. Like Treisman’s, ESP places an emphasis on collaborative problem-solving, though it uniquely also focuses on exploration and discussion of advanced areas of computer science. ESP also closely follows CCNY’s peer-led learning format, where the people who lead the workshops and assist in leading the workshops are undergrads who are just a year or a few years ahead of those in the program (who are typically first-years). So they think, “This could be me down the road if I stick with computer science,” as opposed to being assisted by faculty or even a PhD student, both of whom are much farther along in their careers. The program lives and dies by the dedication, hard work, and quality of our workshop assistants and peer leaders. Moreover, the evidence in the scientific literature as to the efficacy of these types of programs shows that the level of additional student learning is actually incredibly economical.
Another element that makes ESP so successful is that it doesn’t play into the stereotype that computer science is all about programming, which can be a detractor for women and other groups. One of the ways that we hope to be more inclusive and welcoming is by showing that computer science includes tools to tackle social issues like privacy and fairness, because these are important to our students. We work very hard to show them that computer science isn’t just coding, it’s all these other topics as well that we attack with other tools besides just sitting at a computer and typing out some program. It gives them a more complete picture of what computer science is and, hopefully, a more complete picture of what they could do with that knowledge.